Tools like ChatGPT and Dalle-E from OpenAI were quickly embraced by the business and content creation communities. But what precisely is generative AI, how does it work, and why is it such a contentious and hot topic?
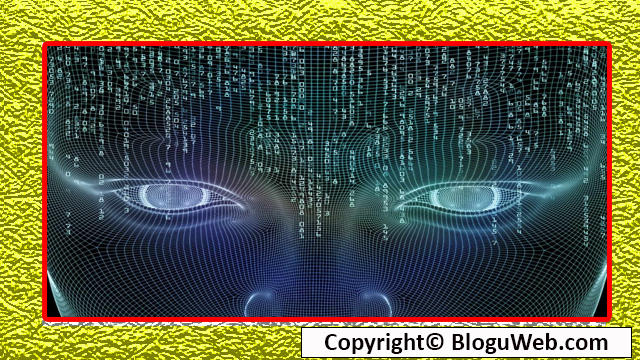
Gen AI, to put it simply, is a subset of artificial intelligence that employs algorithms to create outputs that resemble human-authored text, images, music, graphics, and other types of media.
With general AI, algorithms are developed to learn from training data that contains examples of the desired outcome. By looking at the patterns and structures in the training data, general artificial intelligence (gen-AI) models may produce new material with characteristics similar to the original input data. Information produced by Gen AI may in this way appear real and human-like.
Application of Gen AI
Generative AI is built on machine learning techniques based on neural networks, which are the structure and function of the human brain. During training, a large amount of data is fed to the model’s algorithms, acting as its learning base. Any content relevant to the work, such as text, code, images, and others, may be included in this methodology.
After gathering the training data, the AI model looks for correlations and patterns to understand the underlying ideas driving the content. The AI model continuously modifies its settings as it gains knowledge, improving its ability to mimic content produced by humans. As the AI model generates more content, its outputs become more sophisticated and convincing.
Gen AI has advanced significantly in recent years as a result of various technologies grabbing the public’s attention and stirring up controversy among content creators. Google, Microsoft, Amazon, and other significant IT businesses have prepared their own new generation of AI tools.
Consider ChatGPT and Dalle-E 2 as examples of generation-AI tools that, depending on the application, may rely on an input prompt to guide it towards producing a desired result.
Some of the most notable applications of generational AI tools include the ones listed below.
In response to cues, the AI language model ChatGPT, developed by OpenAI, can produce text that resembles human speech.
A second-generation AI model from OpenAI, Dalle-E 2, creates visual content using text-based cues.
Google Bard is a generation-AI chatbot developed as a ChatGPT competitor and trained on the PaLM large language model.
GitHub Copilot: GitHub Copilot is an AI-powered coding tool that suggests code completions for users of programming environments like Visual Studio and JetBrains. It was created by GitHub and OpenAI.
Midjourney: Similar to Dalle-E 2, Midjourney was made by a San Francisco-based independent research lab. It generates incredibly photorealistic visual data by interpreting linguistic cues and context.
Utilization Cases for Gen AI
While general artificial intelligence is still in its infancy, it has already made a name for itself in a number of industries and applications.
For instance, as part of the content creation process, generation AI might produce text, graphics, and even music, assisting marketers, journalists, and artists with their creative processes. Artificial intelligence-powered chatbots and virtual assistants can provide more individualized assistance, hasten response times, and reduce the workload of customer service representatives.
The following also employ Gen AI:
Medical Research: Gen AI is applied in medicine to expedite the creation of brand-new drugs and lower research expenses.
Marketing: To create customized campaigns and tailor the content to the preferences of their target audience, advertisers use generation AI.
Environment: Climate scientists simulate the effects of climate change and forecast weather patterns using generational AI models.
Finance: To analyze market trends and foresee stock market developments, financial professionals use general AI.
Education: Depending on the learning preferences of each student, some instructors use generation AI models to develop assessments and learning materials.
Risks and Limitations of Modern AI
We must address the issues that Gen AI raises. Its potential to spread false, harmful, or sensitive information that could seriously harm people or businesses and possibly jeopardize national security is a major concern.
These threats have caught the attention of policymakers. In April, the European Union proposed new copyright laws for generation AI, requiring companies to disclose any copyrighted materials used in the development of these technologies.
These laws seek to promote moral development of AI while preventing the abuse or infringement of intellectual property. Additionally, they provide some measure of protection to content producers by guarding against unintentional copying or replication of their work by general AI methodologies.
The spread of automation through generative artificial intelligence (AI) could have a significant impact on the labor force and possibly result in job displacement. Additionally, gen-AI models run the risk of unintentionally amplifying biases present in the training data, leading to unfavorable outcomes that validate unfavorable theories and prejudices. Numerous users frequently overlook this phenomenon because it is an unnoticed side effect.
Since their releases, ChatGPT, Bing AI, and Google Bard have all drawn criticism for their inaccurate or harmful results. As general AI advances, these issues must be addressed, especially given the difficulty of carefully scrutinizing the sources used to train AI models.
It’s concerning when AI companies show apathy.
For a variety of reasons, some tech companies show apathy toward the dangers posed by next-generation AI.
First, they might put immediate financial gain and strategic advantage ahead of long-term ethical considerations.
Second, they might not be aware of or understand the potential dangers of generation AI.
Third, some businesses might disregard the risks because they believe that the government regulations are inadequate or taking too long to implement.
Last but not least, an overly pessimistic view of AI’s potential risks may minimize actual risks while ignoring the necessity of addressing and reducing gen AI risks.
As I’ve mentioned in previous posts, I’ve noticed senior leadership at several tech companies to be almost startlingly dismissive of the risks of misinformation posed by AI, particularly by deep fake images and (especially) videos.
Furthermore, reports have shown that AI has imitated loved ones’ voices in order to demand money. Since many silicon ingredient suppliers are confident that these AI-generated content disclosures will be minimized or ignored, they seem content to place the burden of AI-labeling on the device or app provider.
Some of these companies have expressed concern over these risks, but they have sidestepped the issue by claiming that “internal committees” are still debating their specific policy stances. The fact that many of these businesses didn’t have explicit policies in place to help detect deep fakes didn’t stop them from releasing their silicon solutions on the market, though.
Seven AI leaders consent to voluntary standards
On the plus side, the White House announced last week that a group of voluntary standards for ethical and transparent research have been accepted by seven significant artificial intelligence actors.
President Biden addressed the audience as he welcomed representatives from Amazon, Anthropic, Google, Inflection, Meta, Microsoft, and OpenAI. He emphasized the duty these companies have to take advantage of the enormous potential of AI while doing everything in their power to minimize the significant risks.
The seven businesses agreed to test the security of their AI systems both internally and externally before releasing them to the public. They’ll exchange data, give security investments top priority, and develop tools to aid in the identification of content produced by AI. In addition, they seek to create strategies that could deal with the most urgent problems facing society.
The most well-known global silicon companies were noticeably missing from this list, despite the fact that it represents a positive step forward.
Thoughts for the end
To protect people from the risks of deeply fake images and videos, a multifaceted strategy is required:.
Technology advancements must concentrate on creating reliable detection tools that can recognize complex manipulations.
Campaigns for widespread public awareness should inform people about the existence and dangers of deep fakes.
In order to establish standards and regulations for responsible AI use, cooperation between tech companies, governments, and researchers is essential.
Individuals can gain the ability to distinguish between real and fake content by developing their media literacy and critical thinking abilities.
These efforts can be combined in an effort to safeguard society from the negative effects of deep fakes.
All silicon companies would then be required to develop and provide the necessary digital watermarking technology so that consumers could use a smartphone app to scan an image or video to determine whether it was produced using artificial intelligence. This would be the final step in establishing public confidence. American silicon companies need to step up and take the initiative on this, rather than dismissing it as the responsibility of the device or app developer.
Since it can be easily removed or cropped out, traditional watermarking is insufficient. Even though it is not foolproof, a digital watermarking strategy could alert users with a reasonable degree of confidence that, for instance, there is an 80% chance that an image was produced with AI. This action would represent a significant step in the right direction.
Unfortunately, until something egregious happens as a result of generation AI, such as people getting hurt or killed, the public’s demands for this kind of common-sense safeguard, whether government-ordered or self-regulated, will be ignored. I really hope I’m wrong, but given the competing dynamics and “gold rush” mentality at work, I think this will be the case.